The mathematical models are made at different levels and for different types of applications. Creating a model means observing a process or a phenomenon and trying to understand, e.g., its fundamental biological, physical, or chemical laws, translating them into equations (this is the part of modeling) and then using the computer to solve them. Subsequently, depending on the context, different approaches are possible.
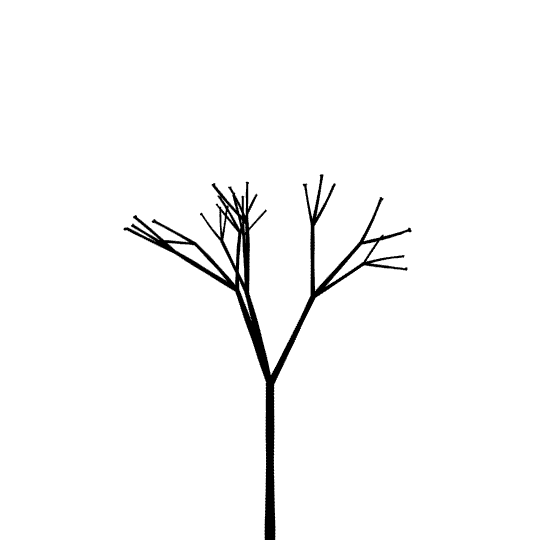
When modelling amorphous structures, for example, we typically use the physical laws to generate the equations of the model, and we can obtain certified, precise, clear, reliable and deterministic answers. When, instead, we deal with living structures – be they plants, animals or human beings – a lot changes. In this case, there is never a deterministic behavior: a subject, depending on his emotional or physiological state or environmental conditions, may react differently to the same stimulus. Making a model means trying to reproduce these processes in a deterministic way, at least for those parts that can assume a deterministic value, possibly calculating all the fluctuations that can characterize the reactions to the process. In a deterministic process, therefore, there is a cause-effect / stimulus-reaction relationship that is not observable in a non-deterministic process, due to changing conditions that can influence the process itself. In a non deterministic model, the quality of the response is not certified as in a purely deterministic model. This implies that the use made of the model is also different: the model has an enormous descriptive capacity, but it is important to understand why it is being used. Depending on the answer one wants to obtain, it is possible to modify it, simplify it, enrich it, so that the answer obtained is conditioned by the question that is being asked. Living beings are made to carry out many processes, as demonstrated by the fact that they perform different functions with different objectives. The human being is not a deterministic machine programmed to give a certain type of response. Depending on the answers to be obtained, the model will then be tuned, enriching or reducing it (i.e. simplifying it).
It is therefore important to emphasize that it is not possible to have universal models that can describe a living being in its extreme complexity, because there is too much variability. The choice of the model is therefore driven by the specific question to be answered.
Translating this into practice, if a model is used to describe the cardiac function and the interlocutor is a cardiologist, interested in heart rhythm, the model must be able to provide answers concerning the reconstruction of cardiac potentials. If the interlocutor is a cardiac surgeon specialized in valves, the electrical component will be of minor importance and the model should instead be able to predict which are the loads of the blood fluid on the valve (therefore, fluid component), or the deformation of the myocardium (hence the mechanical component). If, on the other hand, a coronary bypass has to be realized and blood has to be brought to a necrotized area, the model must be able to describe the perfusion of the myocardium. It is therefore illusory to think of having a model that can do everything, because each model has its own performance criteria. There is therefore no certified and universal model, capable of giving answers to all these possible questions and, if it existed, its cost would be very high: the resources useful for its resolution would be considerable. Today, to simulate a single heartbeat, Europe’s largest supercomputer solves equations for several hours. This is a very important variable to consider, especially if the interlocutor needs quick answers, as often happens in the medical and surgical field. For this reason, sometimes, a model tuning is performed to provide answer in an acceptable time. It is therefore necessary to reduce the complexity of the model, or to simplify it. There may also be models with different complexity, capable of operating simultaneously.
That’s exactly what iHEART is aiming for. For example, when describing a carotid artery, to understand if it could be subject to an occlusion and therefore not to guarantee a sufficient blood supply to the brain, a very refined model must be used, because pressure, forces and velocities must be simulated. Clearly, it is out of reach the use of the same model for the description of the whole cardiovascular system: for example, for the distal circulation in the capillaries one could devise a much coarser model, while more refined models will be used to observe specific locations the researchers are particularly interested to.Intermediate scale models can be used to describe and simulate situations that lie halfway between these two opposites.
Data are essential to “feed” the model. Data together with the model generate information, but data without a model based on first physical principle have a much lower potential. Extracting information from data, without knowing or disregarding the process that has generated them, can lead to considering correlations totally devoid of true statistical value. So, even thinking about the application of models in the medical or surgical field, having a model capable of giving that specific answer someone is looking for is much more important than having a huge amount of data and sticking on them. In addition, a complex and integrated model can lead the interlocutor to ask questions that would never have been otherwise posed, thus opening the doors to new areas of knowledge.